|
Remember that Analytics does not apply changes to historical data, so the moment we assign that value of it is as if we were starting from scratch we will take action and begin segmenting as I anticipated at the beginning of this point. Now we can segment by microbehaviors or traffic sources. To put it simply: what is the probability of gaining a subscriber from SEO, Facebook or Twitter ? And on that note, how much is each SEO, Facebook or Twitter visit worth? Let's go deeper and segment web users into those who view between and pages on average, or between and , and finally more than Which range has a greater propensity to subscribe?
Can you improve the blog to drive a greater number of page views if you Special Database detect that this affects subscriptions? What topics generate the most subscribers? What value does having an internal search engine bring us? Having set a reference value of to get a subscription, we will begin to see in our filtered reports that visits from Facebook have a value of , but from Twitter a value of , and from Linkedin a value of (making up the numbers ). Going into more detail, you will see that women who come from Twitter have a value of and men have a value of , but on.
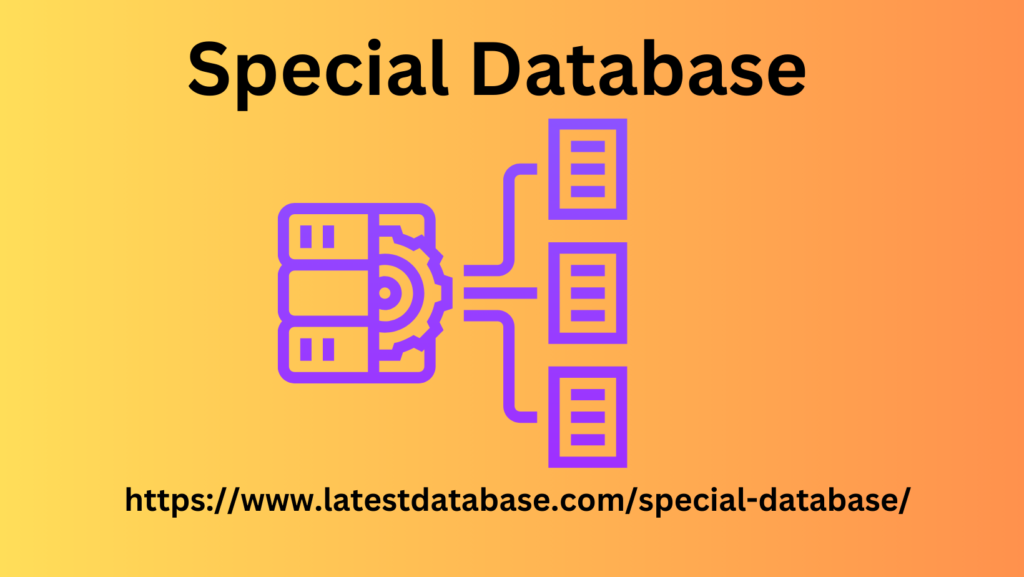
Linkedin, men have a value of , and if they are between and years old, their value increases to Don't you think that this information is beginning to give you very powerful data to improve the performance and strategy of your blog? A step further would be those who have guides to download on your blog, since they surely have a greater value than the blog subscription. In this case, the value ceiling will be set by the guide, we would eliminate from the objective of “being a subscriber”, and we would let that value regenerate naturally based on how it contributes to the download of guides (as long as that is your objective major). If it were not, you can do the reverse analysis and let the guides provide subscribers if both objectives are not connected.
|
|